Generative AI sees the "Unseen"
- Sayantan Auddy
- Apr 23, 2024
- 2 min read
Updated: Apr 23, 2024
The key to studying the Cosmos lies in understanding the observed structures in the interstellar medium, like galaxies, Nebula, Stars and Planets. Astrophysicist compares such observations with simulated models to infer the history of formation and evolution of these celestrial bodies. One such structure of interest is Protoplanetary disk (PPD).
Protoplanetary disks, commonly known as the "birthplaces of the planet," are remarkable formations showcasing the initial phases of planetary system development. These disks are primarily made up of gas and dust, leftovers from the creation of the central star. Serving as a storage space, the disk contains the substances that will later merge to form planets, moons, and various other cosmic entities.

Figure 1: Gallery of observed Protoplanetary disks
PPDs although frequently observed with telescopes such as ALMA are usually oriented at random angles in the three dimensional sky (see Figure 1). They can have an inclination along the line of sight as well as a rotation in the plane of the sky.
It is prefered to observe such disk like structures in its face-on orientation as that provides maximum viewing angle and thus most information. However, the random orientation (inclination and rotation) along with large distance (over ~100s of lights years) from Earth makes it difficult to know their actual structure (face-on). Thus studying such systems are hard and prone to error.
Generative models like GAN (Generative Adversarial Network) enable us to generate face-on images of PPDs from randomly oriented PPD images. This enables researcher to promptly analyze these images, a process that would otherwise demand costly simulations or rough approximations. This is the key to constraining disk properties better and thus help in our understanding of the fundamentals of Planet formation.
We adopt a GAN architecture to generate face-on image for a given randomly oriented image. The GAN model is trained on 1000s of simulated images, where we feed the randomly oriented image as input and network is made to generate a face-on image see Figure 2.

Figure 2. GAN Architecture used to rotate the PPD images
Figure 2 depict how the GAN model rotates the protoplanetary disk image from any arbitrary orientation to face-on image. We adopt the UNET-based PIX2PIX architecture. Additionally we also include attention along with the skip connection (for details check-out the manuscript (in preparation))
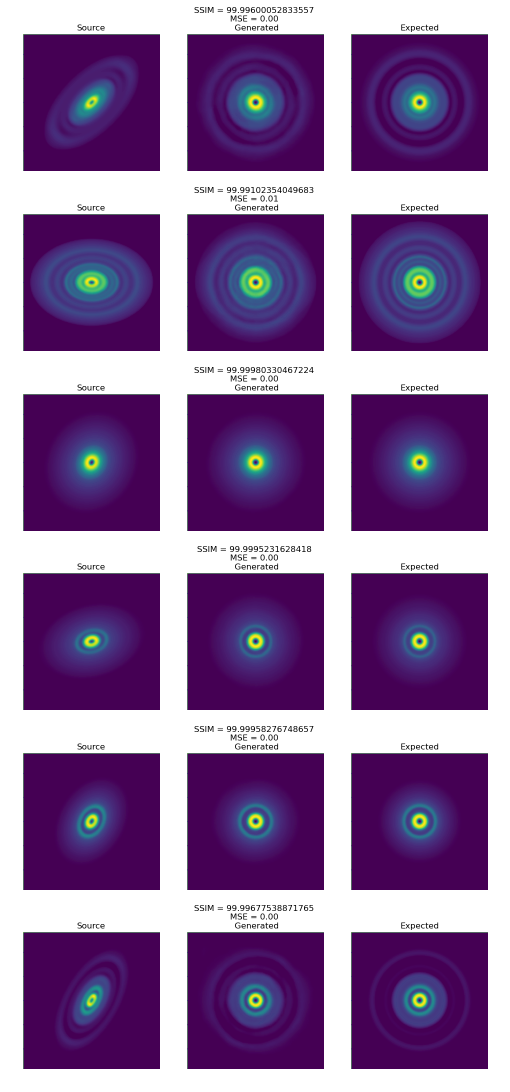
Figure 3 demonstrates example cases, where the trained GAN model rotates the randomly oriented input image to generate a face-on version. Thus our trained model is generating new pixels (information) from limited visible data and we can essentially see the "unseen" . The last column shows the expected face-on image. The SSIM score gives a comparison matrix on how close the generated output is to the expected result. A SSIM score of 1 shows perfect match.
Once the model is trained we test the model with actual ALMA images. The initial results are fascination. Currently the manuscript is under preparation.
Figure 3: Rotated images with GAN
Github code: https://github.com/sauddy/Generative_Models
Our trained model will be made public for other to feed their observed images and generate the necessary face-on image.
Stay tuned for further updates. The paper is under preparation. This work is done in collaboration with Dyutiman Santra and Ramit Dey.
Comments